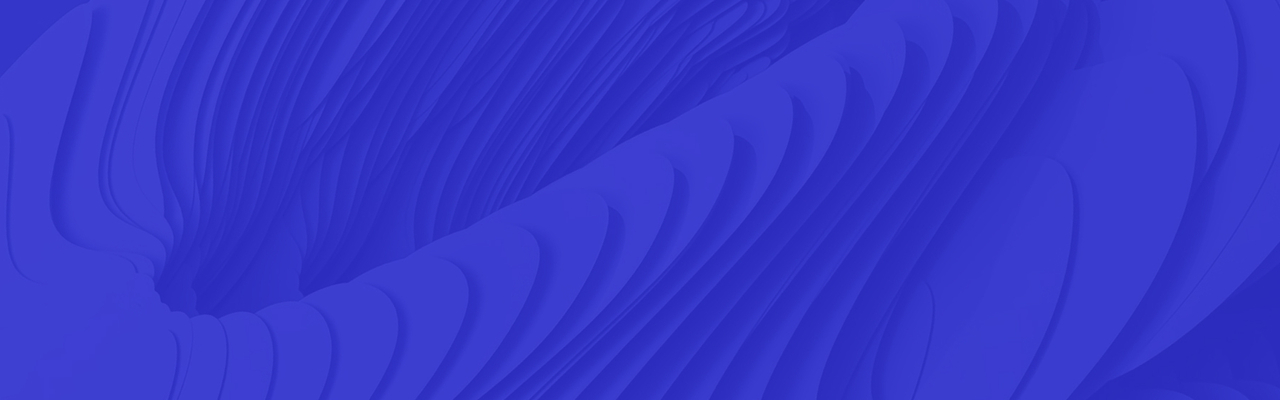
A master data strategy for RegTech
If every financial institution were to implement a master data strategy as part of their RegTech strategy, this would result in a financial system that is more transparent, stable and cost-efficient.
The Value of Data
The acquisition of data has become the gold rush of the 21st century. But as with valuable resources, those that control it tend to be more concerned with preventing access to it than sharing it. However, there are some instances where sharing this resource can be more valuable than protecting it. Data has one key aspect that differentiates it from gold or oil. It can be duplicated an infinite number of times and shared. This means that if 2 people each have a piece of valuable data, and they share it, they will each have 2 pieces of data (i.e.. 1 + 1 = 4), generating an extra value of 2.
The two critical assumptions to mention here are that in order to encourage this sharing, (a) both parties must be interested or value the other parties’ data and (b) the cost of sharing must be dramatically less than the value it generates. Unfortunately, in some cases (such as finance) where data has always been a key component of business, data is often complex, poor quality, outdated and stored in a myriad of different forms and places. In these cases, the cost of turning that data into a shareable form would require a considerable ETL process that can cost many millions. So costly in fact, that even the 2x return in the example above is not enough. But if instead of 2 parties, you had 1000, then suddenly the return on sharing would be 1000x, which could be very worthwhile. This is where a data standard can play an immense role to generate value. If every financial institution were to implement a master data strategy as part of their RegTech strategy, this would result in a financial system that is more transparent, stable and cost-efficient.
Industries like transportation and healthcare have seen massive benefits from sharing data to make everyone safer and healthier. This is not to mention the ‘Data Giants’ such as Facebook and Google, who in just over a decade have turned data into a commodity that is on par with oil. However, it is also important to realise that if the data is not refined and converted into a usable form, then it cannot be exploited. The value of data lies in the analytical tools, and increasingly in machine learning and AI technologies, that can be applied to it. While the Tech Giants realised this many years ago, the financial industry is yet to do so. Given the pace of technological progress in recent years, we have come to a point where the technology already exists to solve the big data problems of financial institutions, but what is missing are the data standards necessary to facilitate these tools.
Lessons from the Crisis
The financial crisis brought into focus both the global scale of the financial industry as well as the fragility of such an interconnected network without the correct supervision. If the banks in 2008 had been motivated to implement a master data strategy in their internal systems, then we probably would have seen that the industry was about to explode. For example, if a common data format for mortgages had existed in 2007, banks could have seen growing risks in mortgage-backed securities more readily instead of just relying on top-level ratings. The financial crisis brought to light the inadequacy of the systems and standards used to measure financial data pre-financial crisis. This lies in stark contrast to the level of integration that an effective strategy should achieve.
Before the crisis, financial institutions only worried about a one-dimensional flow of regulatory data, where data would flow from front, to middle, and to back office, and finally it was used for regulatory purposes. In order to facilitate this flow of data, scripts upon scripts would be piled on top of each other, and regulation was an afterthought.
One of the primary reasons why these systems failed was because a common data format by which to identify and record financial transactions has not existed for the industry. While every financial institution is different in its own way, regulations require every firm to collect certain data points with specific definitions. As these requirements have evolved and developed over time, no comprehensive documentation exists for what granular data needs to be collected. It follows that if you want to start a new bank and issue a mortgage, there is no readily accessible technical documentation for all the fields you need to record for a mortgage. As such, every firm, and various functional units within that firm, collects and stores this data in a different format with different definitions (or worse, relies on their vendor to decide for them). This makes data exchange between, firms, firms and regulators and internal IT systems a manual and error-prone ETL process. As the financial industry boomed in the nineties and into the twenty-first century, and billions of financial transactions were ingested into these error prone systems, the risks that this posed eventually came to light in an abrupt and unforgiving fashion.
The Spirit of the Regulation
In the wake of the financial crisis, the financial world was hurled through a regulatory overhaul. Financial institutions have been forced to expend an increasing quantity of time and resources to fulfil the financial regulator’s demands.
Regulation is often perceived as costly, time consuming, and a hassle. However, by looking further to the ‘spirit’ of the regulation, what the regulator is ultimately seeking from industry incumbents is discovered. This can be answered in one word – transparency. It has become increasingly clear from post-crisis financial regulation that the regulator wants to be able to collect and monitor more financial data, more frequently, and at a higher level of granularity. What is also important to realise is that by doing this, inadvertently, the regulator is compelling the industry to eradicate the legacy systems that still exist internally in many systemically important financial institutions. The post-crisis regulator is no longer willing to tolerate the lack of transparency and auditability of these black box systems.
In the era of regulation, the winners will be the institutions who accept and are empowered by the regulator’s demands, and not those who shy away from this challenge and continue to throw money at outdated systems. If we recall the one-dimensional flow of data outlined above, in stark contrast financial regulation is now requiring banks to accommodate data flowing from one system to another, and then from one department to another. This is where antiquated systems where scripts are piled on top of each other simply will not work, or if they do work, they will incur excessive costs. Furthermore, the crisis and regulatory weight has left banks bordering on profitability, no longer having the deep pockets and resources to support complex, bespoke, internal systems. Standardisation of non-core functions like reporting and data storage will help reduce costs dramatically across the industry.
This is why a master data strategy, driven by intelligible data standards, is an essential element of a RegTech strategy. Internally, the right data strategy allows for proper control and governance of key data points and a comprehensive understanding of core regulatory fields and supplemental data fields that are proprietary to a firm. Converting to a common data format allows firms to fill in the gaps in their regulatory data requirements. Externally, it offers regulators comparability of transaction data (not just top-line information), easy exchange of risk and product information amongst firms as well as well-defined integration of systems that use this data.
Developing a Master Data Strategy
A good data standard should be freely and easily accessible to its target community. It should be capable of being used and understood by anyone working with financial data without the need for any 3rd party software licenses. Moreover, the documentation supporting the standard should be user-friendly, clear, concise and understood by a wide audience. A most crucial requirement on this topic, is that standards must be technologically digestible. For example, a developer who knows nothing about finance should be able to consume the data standard and create technology using that standard.
Another requirement is that of neutrality. The data format needs to capture data in a way that is not specific or tailored to a certain business model, vendor or financial institution. Regulation is applied evenly across the board to all firms. Furthermore, deciding to focus on regulatory data provides a mechanism for being very clear from the outset on the objectives and primary use-cases for the format, helping to avoid disjointed development efforts pulling the project in different directions. A new project such as the development of a new data standard cannot afford to not know what it is doing, or it will be doomed to fail.
Even with clear priorities, difficult decisions have to be made and there are best practices that can be employed in order to ensure stable development of a data strategy and a more successful adoption. One decision to be made is whether the standard will be a standard by nature or a standard by adoption. True standards are carefully crafted by experts, such as ISO or W3C, after much deliberation and organised consultation with a panel of specialists. Other standards come into being in a more eventual manner through continuous development and gradual acceptance by market practitioners. Clarifying the development approach is an important question for potential users and the community and should be included in the project’s scope. As adoption increases, it is possible to move to a more formal approach and share the operational overhead with other key stakeholders. That being said, it is important to not put off the formal process for too long as it can lead to a project losing focus and/or not fulfilling its objectives.
In addition to this, planning on dealing with practical realities is a must. Starting from a blank page, a standard has the luxury of defining an ideal solution. Practically however, the project’s key principles can become compromised when faced with considerable challenges to implementation. For example, financial institutions often have gaps in their data which means a ‘perfect’ data schema might be impossible to implement. Dealing with issues like these requires careful assessment of the pros and cons of the compromise and must be done on a case by case basis. One of the principles of the Open Stand organisation is to “address broad market needs” and therefore it can be justified to prioritise usability over theory. Having a formal process and a board of stakeholders can really help decision making for these kinds of issues.
Considering how the data standard will be used is key to the development process. It is important to understand how the schemas will be used from a practical and development standpoint. A good suggestion is to maintain the ‘latest’ version in the same namespace and when a new release is ready, to freeze that release with its own namespace ie. v1.0, v1.1 etc. How the standard will be used will also drive the supporting work that needs to be done such as presentations, documentation, examples and test data.
Finally, the creator of a data format should not be afraid of building on what is already available. It is likely that many intelligent and talented people, both inside and external to the creator’s organisation, have done related or correlated work in the same field. Ignoring their results would be a huge waste of a valuable resource, particularly if they have tackled a large problem.
The Financial Regulatory (FIRE) Data Standard
In Jan 2016, following 18 months of research into regulatory data, Suade was awarded a grant supported by Tim Berners-Lee’s Open Data Institute and the European Commission to develop a regulatory data standard for the financial industry.
The Financial Regulatory (FIRE) Data Standard was designed, developed and published to achieve many of the goals set out in this paper. FIRE has three core tenets: easy to understand, easy to use and open.
To achieve ease of understanding, the project was developed to be read and consumed by those familiar with the subject matter, but without technical programming knowledge, as well as programmers without any kind of financial background. Most of the project is simple text files with informal descriptions, examples and educational information scattered throughout. For ease of use, it was decided to host the project on GitHub, the top site for collaborative open source projects, with a mirror site hosted by Suade. Moreover, the design and layout of the schemas and their cross-dependencies was also implemented in such a way that could be practically implemented by today’s financial institutions, keeping relational concepts and building off of other well known ISO and industry standards. Finally, as an open source project, the schemas, documentation, validation engine and examples are all free to use, modify and distribute under the Apache 2.0 open source license.
To this day, it remains the only open source, free, data standard available for the transmission and storage of financial data. As of 2019, over 500 firms were subscribed to the project.
From Master Data Strategy to RegTech Strategy
The Financial Conduct Authority defines [RegTech][regtech] as follows: ‘RegTech applies to new technologies developed to help overcome regulatory challenges in financial services’. Arguably the most significant element of this definition is the concept of ‘new technologies’, and this re-enforces the notion that outmoded systems for processing financial data should have no place in any successful RegTech strategy. Over the past few decades there has been a phenomenon of technological disruption across many industries and the financial industry has capitalised on these opportunities. In recent years many innovative technologies have creeped up on the industry and proved their ability to do things cheaper and faster.
Technology has undoubtedly raised the bar, and therefore a data standard which is not adaptable and scalable to the current pace of technological development will quickly become a critical hurdle in a RegTech strategy. This follows from the fact that standards are of utmost importance in any form of technology. For example, the internet’s foundations were laid in the late 80s and early 90s through a set of fundamental standards and are still governed by a few key organisations. Key to this success has been the adoption of basic HTML formats and standards for sending and receiving data. When contemplating a RegTech strategy, a key factor is that standards are what make software possible. Every time there has been a huge leap in the way that we transfer data it has been through a new standard. Now that we are in the era of regulation, it follows that regulatory data standards are at the fulcrum of the transition to a RegTech strategy.
Implementing a data standard as part of a Regtech strategy also enables firms to escape from vendor addiction and instead allow the technology to come first, while simultaneously reducing costs and promoting innovation within the business. The regulator is demanding a level of interoperability that will not tolerate a situation where a firm needs one vendor’s solution to make a submission. Another vital element of a RegTech strategy is open, API driven architecture. As stated above, transparency is what the regulation is forcing industry players to achieve, and therefore any systems which you cannot programmatically interrogate is essentially another legacy system. It is a knot in the matrix of data that must be able to move from one place of the network to the other with ease.
Going beyond Regulatory Compliance
At present, there is a reality where financial institutions are hoarding an abundance of financial data yet given the epidemic of big data issues that most financial institutions currently face, the value of this data is never actualised. However, at the same time, the regulator is obliging industry incumbents to straighten out their data management methodologies. The champions in the age of regulation will be those who follow the spirit of the regulation and assume a RegTech strategy which will essentially free financial data from legacy systems and poor data management techniques. In doing this, the firms that are victorious will also find the answer to many of their big data problems. This opens up opportunities that go far beyond regulatory compliance, and at this point the potential of applying revolutionary technologies such as artificial intelligence and machine learning to financial data can be achieved.
As such, a master data strategy is a fundamental starting point for creating a RegTech strategy that is future proof and will present rich opportunities to avail of emerging technologies.
[JUNE 2019]